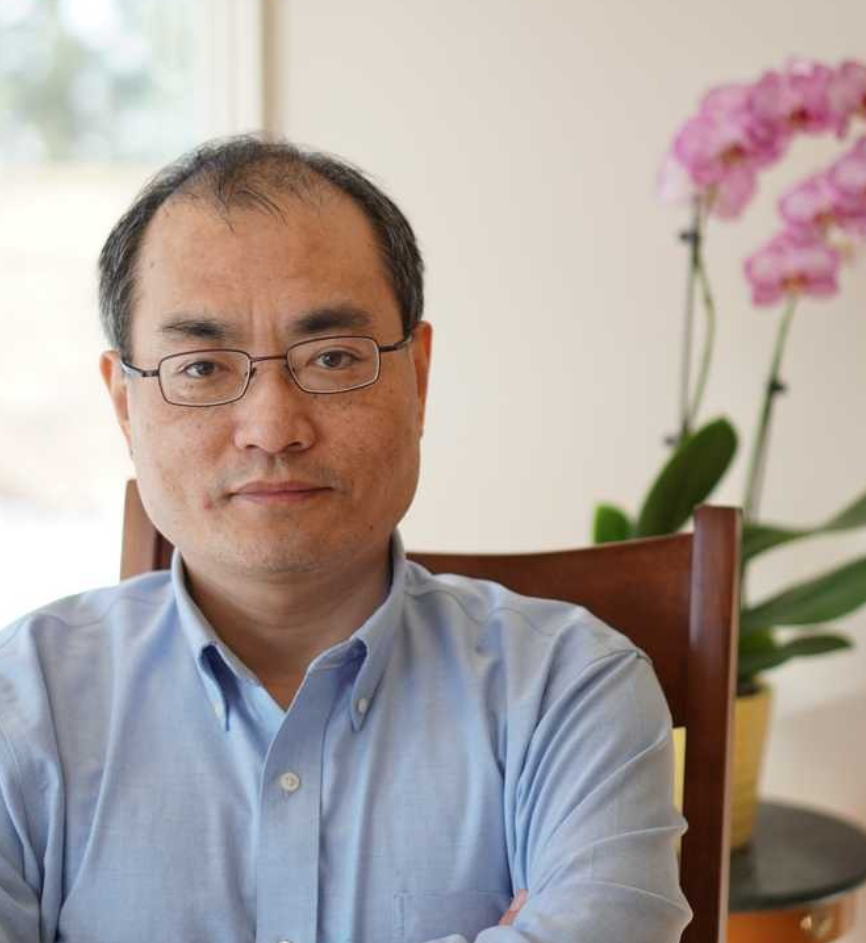
The root of all innovation, the pinnacle of all great advancements, the motivation for everything to come, may lie in the hands of the 21st century’s most crucial resource: data. Advances in computing and communication technology, along with the growth that has occurred in the field of data science and artificial intelligence, have put society in a position to truly leverage data in unprecedented ways, impacting how we interact with our surroundings on a daily basis.
Two well-respected UW-Madison researchers stand in the midst of this movement, using the technologies of today to create what were once the ideas of the distant future. Dr. Raj Veeramani is the E-Business Chair Professor and holds joint appointments in the department of industrial and systems engineering in the College of Engineering and the operations and information management department in the Wisconsin School of Business. Dr. Shiyu Zhou is a Vilas Distinguished Achievement Professor of industrial and systems engineering. Their research focuses on applying data science and machine learning techniques to improve business processes and automation.
Rapid advances in computing and the sheer interconnectedness of society have created implications for our everyday interactions that are the focus of today’s research. Everything from sensors in a factory machine to how we as consumers interact with businesses, both directly and indirectly, creates observable data for companies looking to improve decision making, machine productivity, and the overall business processes. Combining this data with decision science is the heart of Dr. Zhou and Dr. Veeramani’s research. Conventional thinking suggests that data is purely composed of statistics and data that can easily be plotted on a coordinate plane. However, the existence of (and more importantly the handling of) unstructured data is one of the more advanced practices in the field of data science and machine learning. Comments, maintenance notes written by a technician, remarks written by a customer service representative are just a few examples of “the rich and diverse set of data which are now available and cannot simply be modeled and studied like structured data,” notes Dr. Veeramani.
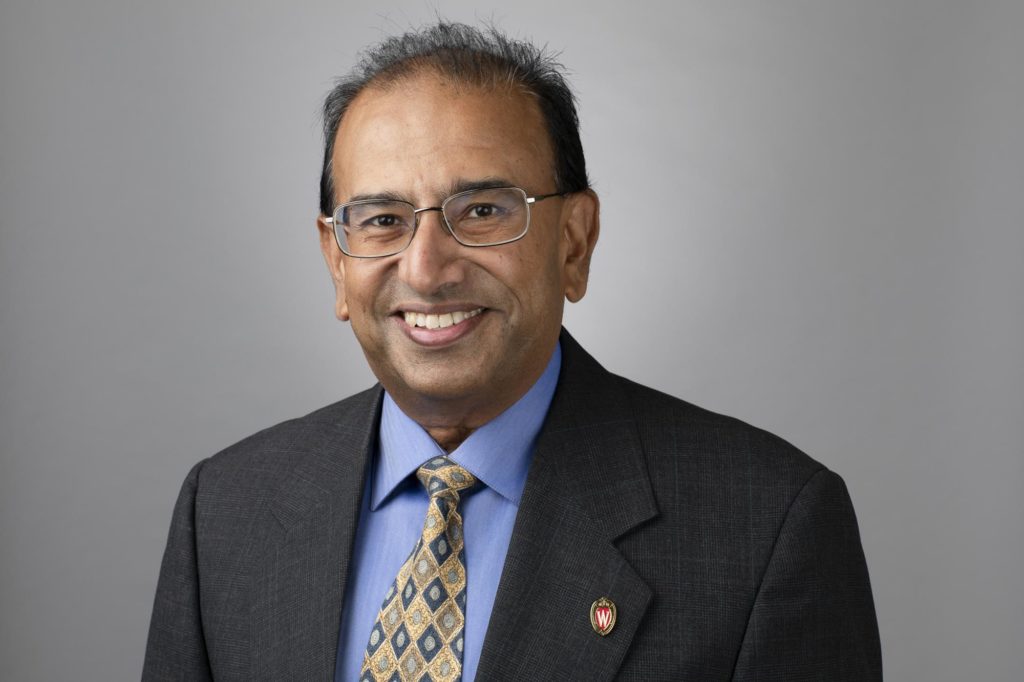
Understanding unstructured data is important because it provides valuable insights to further enhance the capabilities of machines. Machines are primarily built on understanding large quantities of data and improving pre-existing models and calculations, hence the term machine learning. Building relationships between different variables and categories of data, as Dr. Zhou notes, “allows for the creation of models that predict future behavior,” thereby limiting error and improving business performance.
What goes into a given research project can vary depending on not only the kind of data Dr. Veeramani and Dr. Zhou are using, but also on the models that are already in place to address industry concerns. It’s therefore vital for researchers in the field of data science and machine learning to begin with a specific problem that is yet to be addressed and then scale up such that it can reveal a greater industry concern. Talking to industry engineers might, for example, uncover existing problems with a machine coolant or belt functionality that is dramatically impacting production. This discovery then starts the process that eventually uncovers greater inconsistencies throughout the general industry, often requiring “newer models or building on existing principles to answer bigger questions,” Dr. Zhou says.
The challenges that Dr. Zhou and Dr. Veeramani face after addressing a particular problem, however, are far greater than the plethora of information that must be analyzed. Understanding the data from an analytical standpoint poses a challenge in and of itself, because as Dr. Veeramani points out, “analysis isn’t as simple as having an x-y coordinate and creating a model to show correlation [because there are simply too many] aspects that can influence a particular data set.” Simply having access to data isn’t enough to conduct thoughtful research, because the observations could be telling a story that isn’t particularly relevant to the issue at hand. Compensating for the “heterogeneity and oftentimes the incompleteness of data,” as Dr. Veeramani puts it, is a key aspect of their research. Only by pulling the correct information from what seems like an endless amount of data can one derive valuable insights.
Using “pre-existing models is a beneficial starting point,” as Dr. Zhou points out, but oftentimes there are limitations to such techniques. It isn’t enough to solve problems for a particular issue or for a particular company. The most rewarding aspect of Dr. Zhou and Dr. Veeramani’s research lies in the long term impacts this kind of research can have. “We not only have the capability to understand what HAS happened, but to also anticipate what MIGHT happen,” Dr. Veeramani says. “Our aim is to extend the state of the art. To do things that could not be done before.”
Photographs provided by Dr. Zhou and Dr. Veeramani